背景
强化学习,特别是深度强化学习,在近年来取得了令人瞩目的进展。除了应用于模拟器和游戏领域,在工业领域也正取得长足的进步。Ray 是一个为了强化学习或者类似的场景设计的机器学习框架,在最近,Ray 合并了在 Kubernetes 上实现 Ray 集群自动伸缩的代码请求。因此,我们希望在本文中介绍这一新特性,以及上游社区采取的设计方案和其中的考量。
相关知识与工作
强化学习
在正文之前,首先简单介绍下 Ray 面向的场景:强化学习。强化学习是机器学习方法中的一种,这一问题可以被抽象为代理与环境之间的互动关系。环境是代理所处的外部环境,会与代理产生交互。在每次迭代时,代理会观察到部分或者全部的环境,然后决定采取某种行动,而采取的行动又会对环境造成影响。不同的行动会收到来自环境的不同反馈(Reward),而代理的目标就是最大化累积反馈(Return)[1]。
其中,代理可以采取的行动的空间(Action Space)可能是离散的,如围棋等。也可能是连续的。而不少的强化学习算法只能支持连续的空间或者离散的空间。
在采取行动时,代理会根据某种策略(Policy)选择行动。策略可以是确定性的,也可以是带有随机性的。在深度强化学习中,策略会是参数化的,即策略的输出是输入是一组参数的函数(参数比如神经网络的权重和 bias)
强化学习领域有一个非常生动的例子:游戏 Flappy Bird 的 AI [2]。在这一个例子中,代理就是玩家控制的小鸟,而环境就是充满了管道的飞行环境。小鸟的行动空间只有两个动作:向上飞或者什么都不做,原地下坠。同时小鸟的目标就是不断续命,飞行下去。这一问题可以被很好地用强化学习的方法建模解决。具体可见参考文献 [2]。
正文
Ray 架构
在介绍 Ray 如何在 Kubernetes 上实现自动伸缩之前,先大致介绍一下如何使用 Ray。Ray 本身其实并没有实现强化学习的算法,而是一个基于 Actor 模型实现的并行计算库。而诸多高层次的强化学习算法则是由 RLLib 实现。这里为了简化问题,不多讨论 RLLib 是如何利用 Ray 提供的功能实现强化学习算法的过程。
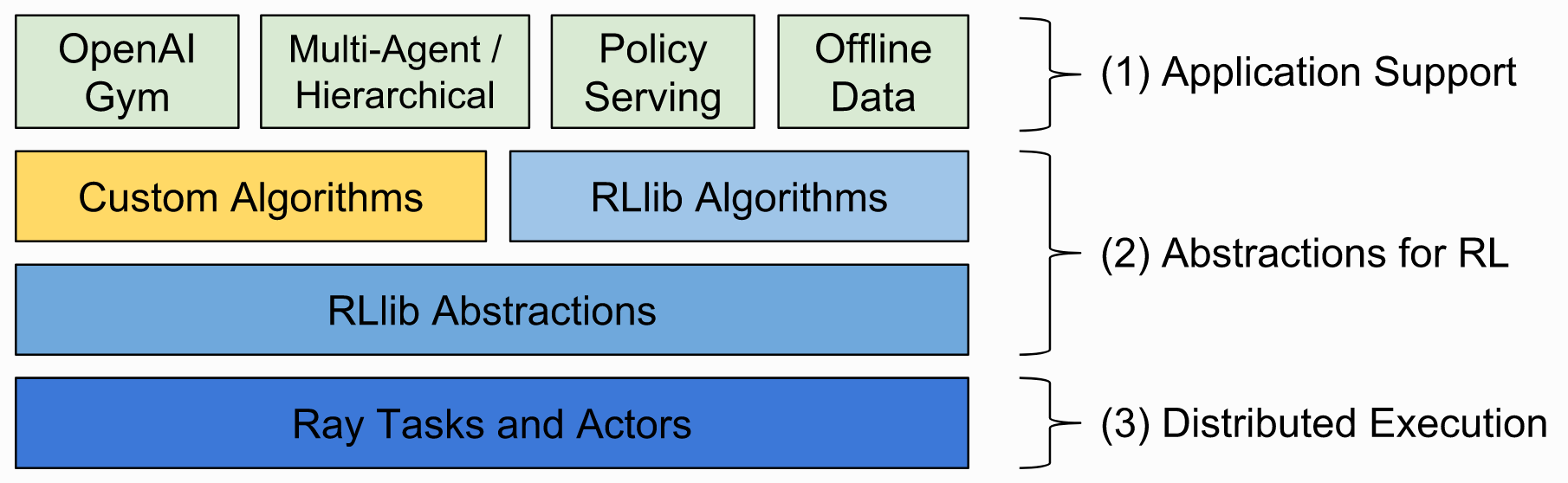
万物先从 Hello World 开始,Ray 下的 Hello World 如下所示。
import ray
ray.init()
@ray.remote
def hello():
return "Hello world !"
object_id = hello.remote()
hello_obj = ray.get(object_id)
print(hello_obj)
这一例子尽管很简单,但用到了许多 Ray 特有的功能。首先,是 @ray.remote
的注解。这一注解的作用是声明这一函数是可以被远程,且异步地执行的。为了实现远程执行,函数的返回并不是在函数中定义的 Hello world!
,而是一个 Object ID(确切地说,是一个 Future 对象),随后创建一个任务(Task),并且会在未来的某个时刻,交由一个 Worker 进程执行,而结果可以利用 Object ID 通过 ray.get(object_id)
获得。
Ray 的论文中有一个 Ray 的整体架构图,但论文发布于 2017 年 3 月份,Ray 开源实现与它相比,有不少出入。这里以代码实现为主,介绍一下 Ray 的一些关键组件。
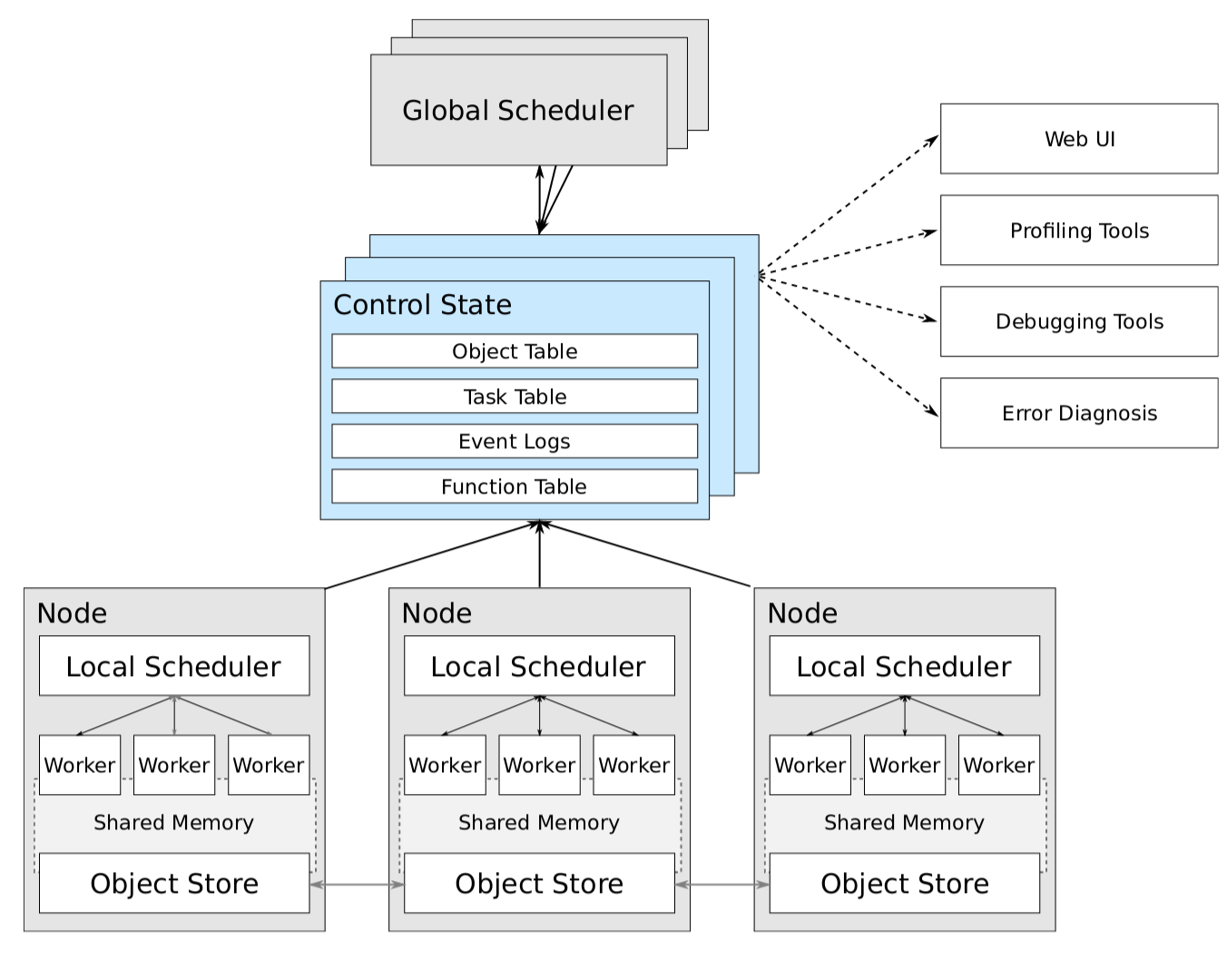
Ray 的节点需要运行两个进程,一个是 RayLet 进程,一个是 Plasma Store(对应图中的 Object Store)进程。其中 RayLet 进程中维护着一个 Node Manager,和一个 Object Manager。Ray 提供了 Python 的 API,而 RayLet 是用 C++ 实现的。其中的 Node Manager 充当了论文中 Local Scheduler 的角色,主要负责管理 Node 下的 Worker,调度在 Node 上的任务,管理任务间的依赖顺序等。而其中的 Object Manager,主要提供了从其他的 Object Manager Pull/Push Object 的能力。
Plasma Store 进程,是一个共享内存的对象存储进程。原本 Plasma 是 Ray 下的,而目前已经是 Apache Arrow 的一部分了。之前介绍 Ray 在执行带有 remote
注解的函数时并不会立刻运行,而是会将其作为任务分发,而返回也会被存入 Object Store 中。这里的 Object Store 就是 Plasma[4]。
而论文中的 Control State,在实现中被叫做 GCS,是基于 Redis 的存储。而 GCS 是运行在一类特殊的节点上的。这类特殊的节点被称作 Head Node。它不仅会运行 GCS,还会运行对其他节点的 Monitor 进程等。
Ray 提交任务的方式与 Spark 非常类似,需要利用 Driver 来提交任务,而任务会在 Worker 上进行执行。Ray 支持的任务分为两类,分别是任务(Task)和 Actor 方法(ActorMethod)。其中任务就是之前的例子中的被打上了 remote
注解的函数。而 Actor 方法是被打上了 remote
注解的类(或叫做 Actor)的成员方法和构造方法。两者的区别在于任务都是无状态的,而 Actor 会保有自己的状态,因此所有的 Actor 方法需要在 Actor 所在的节点才能执行。这也是 Ray 跟 Spark 最大的不一样的地方。Spark 提交的是静态的 DAG,而 Ray 提交的是函数。
Ray 的集群化运行
因此,如果需要一个 Ray 集群,那么一共需要两个角色的节点,分别是 Head 和 Worker。如果是在传统服务器上部署,可以参考 Manual Cluster Setup。而如果是在 Kubernetes 上部署,可以参考 Deploying on Kubernetes。
除此之外,还有一种相对而言更简单的方式,那就是利用 Ray Autoscaler 自动地创建集群。Autoscaler 是 Ray 实现的一个与 Kubernetes HPA 类似的特性。它可以根据集群的负载情况,自动地调整集群的规模。
其需要的配置大致如下:
# A unique identifier for the head node and workers of this cluster.
cluster_name: minimal
# The maximum number of worker nodes to launch in addition to the head
# node. This takes precedence over min_workers. min_workers default to 0.
max_workers: 5
# Cloud-provider specific configuration.
provider:
type: gcp
region: us-west1
availability_zone: us-west1-a
project_id: null # Globally unique project id
# How Ray will authenticate with newly launched nodes.
auth:
ssh_user: ubuntu
用户只需要提供期望的 Worker Node 数量,以及节点资源的提供者(上面的例子中是 GCP),Ray 就会根据负载(默认的阈值是 80%)进行自动扩缩容。
自动扩缩容特性在 Kubernetes 上的设计与实现
自动扩缩容是一个非常具有吸引力的特性。Ray 在之前只支持在 Kubernetes 上运行 Ray 集群,而不支持自动扩缩容。这一功能最近刚刚被实现并且合并到了代码库中。在实现的过程中,Ray 社区尝试了不少思路。
Kubernetes Native 的实现思路
首先出现在社区中的是 Kubernetes Native 的实现思路。社区创建了 ray-project/ray-operator。这一思路有两个探索性质的实现:gaocegege/ray-operator 和 silveryfu/ray-operator。其中前者是定义了一个 CRD Ray
,利用 Deployment 创建 Head 和 Worker 节点。一个最小化的配置如下:
apiVersion: ray.kubeflow.org/v1
kind: Ray
metadata:
name: sample-cluster
spec:
worker:
replicas: 3
header:
replicas: 1
在这样的设计中,可以直接依赖 Kubernetes HPA 进行自动伸缩。但是,这里有一个比较严重的缺陷。Ray 在做 Scale Down 的时候,会根据 Worker 节点的一些状态来判断最适合被回收的节点。一个最直观的例子是要尽可能回收没有运行 Actor 或者没有正在运行的任务的节点。而目前 Kubernetes HPA 不支持如此细粒度的逻辑。在 HPA 的实现中,它是通过操纵 Deployment 的 Replicas 来实现自动扩缩容的。而 Deployment 通过 ReplicaSet 来删除多余的实例。而 ReplicaSet Controller 在删除的时候,通过排序来确定应该删除哪些实例。排序算法本身不具备扩展性,会先从 UnReady 的 Pod 开始,依次删除,直到所有的实例都被删除或者删除到了指定数量。利用 Readiness Gate,可以实现这样的特性,但是首先这一特性比较新,在 1.14 才刚刚 Stable。其次这样的实现方式需要非常多的 Dirty Work,并且比较难以维护和调试。
silveryfu/ray-operator 的实现,定义了两个 CRD,RayHead
和 RayWorker
,同样采取 Deployment 来创建 Head 和 Worker。这样的实现同样具有上述问题。
Ray Native 的实现思路
由于 Ray 本身也有节点的概念,因此 Ray 自身也存在具有一定扩展性的自动扩缩容的实现。既然利用 Kubernetes HPA 很难实现精细化的 Scale Down,那利用 Ray 自身的 Autoscaler 抽象,是否可以实现这样的特性呢?答案是肯定的,社区目前的实现也是如此思路。
在之前介绍 Ray 的扩缩容的时候,提到了节点资源的提供者这样一个概念。这其实就是 Ray 自身提供的,为了支持不同的平台的扩缩容的抽象。任何平台,只要可以实现 Provider
接口,就可以利用 Ray 原生的命令进行集群的扩缩容:
class NodeProvider(object):
"""Interface for getting and returning nodes from a Cloud.
NodeProviders are namespaced by the `cluster_name` parameter; they only
operate on nodes within that namespace.
Nodes may be in one of three states: {pending, running, terminated}. Nodes
appear immediately once started by `create_node`, and transition
immediately to terminated when `terminate_node` is called.
"""
def __init__(self, provider_config, cluster_name):
self.provider_config = provider_config
self.cluster_name = cluster_name
def non_terminated_nodes(self, tag_filters):
"""Return a list of node ids filtered by the specified tags dict.
This list must not include terminated nodes. For performance reasons,
providers are allowed to cache the result of a call to nodes() to
serve single-node queries (e.g. is_running(node_id)). This means that
nodes() must be called again to refresh results.
Examples:
>>> provider.non_terminated_nodes({TAG_RAY_NODE_TYPE: "worker"})
["node-1", "node-2"]
"""
raise NotImplementedError
def is_running(self, node_id):
"""Return whether the specified node is running."""
raise NotImplementedError
def is_terminated(self, node_id):
"""Return whether the specified node is terminated."""
raise NotImplementedError
def node_tags(self, node_id):
"""Returns the tags of the given node (string dict)."""
raise NotImplementedError
def external_ip(self, node_id):
"""Returns the external ip of the given node."""
raise NotImplementedError
def internal_ip(self, node_id):
"""Returns the internal ip (Ray ip) of the given node."""
raise NotImplementedError
def create_node(self, node_config, tags, count):
"""Creates a number of nodes within the namespace."""
raise NotImplementedError
def set_node_tags(self, node_id, tags):
"""Sets the tag values (string dict) for the specified node."""
raise NotImplementedError
def terminate_node(self, node_id):
"""Terminates the specified node."""
raise NotImplementedError
def terminate_nodes(self, node_ids):
"""Terminates a set of nodes. May be overridden with a batch method."""
for node_id in node_ids:
logger.info("NodeProvider: "
"{}: Terminating node".format(node_id))
self.terminate_node(node_id)
def cleanup(self):
"""Clean-up when a Provider is no longer required."""
pass
仔细观察接口,就会发现其实这些接口都可以利用 Python 的 kubernetes client 来完成。
比如 non_terminated_nodes
,这一接口的返回是当前集群还在运行的节点,我们可以获得 Ray 所有的实例 Pod,进而排除 Failed/Unknown/Succeeded/Terminating 的 Pod 获得。
def non_terminated_nodes(self, tag_filters):
# Match pods that are in the 'Pending' or 'Running' phase.
# Unfortunately there is no OR operator in field selectors, so we
# have to match on NOT any of the other phases.
field_selector = ",".join([
"status.phase!=Failed",
"status.phase!=Unknown",
"status.phase!=Succeeded",
"status.phase!=Terminating",
])
tag_filters[TAG_RAY_CLUSTER_NAME] = self.cluster_name
label_selector = to_label_selector(tag_filters)
pod_list = core_api().list_namespaced_pod(
self.namespace,
field_selector=field_selector,
label_selector=label_selector)
return [pod.metadata.name for pod in pod_list.items]
其他接口也有类似的实现。通过这样的方式,用户可以利用 Ray 原生的命令,实现在 Kubernetes 集群上的扩缩容,具有非常统一的用户体验。
结
本文介绍的内容到这里就结束了,不过值得深入的内容仍然有很多。Spark 目前可以直接利用 Kubernetes 作为 Executor 把 Spark 应用利用 spark-submit
提交给 Kubernetes 执行。而 Ray 是否也可以做到这样的方式呢?Ray 本身依赖着 GCS,和运行在各个节点的 Object Store,这种按需执行的方式无疑比 Spark 要更加复杂。而且与 RLLib 的兼容性也是一个问题。目前 Ray 自身有着 SCRIPT_MODE 这样一个模式。在这样的模式下,可能是可以尝试的。除此之外,Kubernetes Native 的实现在不需要自动扩缩容的场景下,是否具有一定的优势?这里不再展开讨论了,Ray 作为 RISE Lab 新推出的系统,从设计而言,还是有非常多值得称道之处,希望能够在后续文章中再一起探讨。
参考文献
- [1]: Part 1: Key Concepts in RL - OpenAI
- [2]: 带你走进强化学习-Flappy Bird游戏自学习 - 知乎文章
- [3]: Ray Walkthrough
- [4]: The Plasma In-Memory Object Store
License
- This article is licensed under CC BY-NC-SA 3.0.
- Please contact me for commercial use.